AI is, apparently, either the answer to all our problems, our future replacements in all technological occupations or the next .com industry, depending on who you ask. As described in Paul Kennard’s story on AI in HeliOps #152 issue, it’s a bit more nuanced than that. For those of us working in the cockpit of the industry that is aviation, how do we sift through the products on sale? At the moment, there is an expanding portfolio of apps, programs, and devices that are' powered’ by AI to carry out their roles. Not all of them are up to scratch, and in the same way that makers of eVTOL vehicles are sometimes finding out the hard way, aviation regulation is a conservative nut to crack. This is justifiable when incorporating new technology into the cockpit – many of those regulations are written in blood, after all. To identify which products are going to be of benefit to our operation today, and which ones are maybe a few generations away from being used in the cockpit, we can ask a few questions and use one case study of how it has been done right.
Questions
Question one has to be, is it compliant? If the relevant authorities have not deemed the product acceptable for use in the cockpit, there is little point in proceeding. Is it reliable? Is it safe? Does it do something that improves efficiency and safety or otherwise brings value to my flight? Currently, AI products are usually used in the less safety critical parts of aviation. Rostering, customer-facing chatbots and dynamic ticket pricing are all areas where AI-based products have simplified user experience to the point of providing oversight and approval rather than having actually to do the work. In the cockpit, however, consequences of errors are more likely to manifest themselves kinetically. Let’s take a scenario to see how an existing product can actually help.
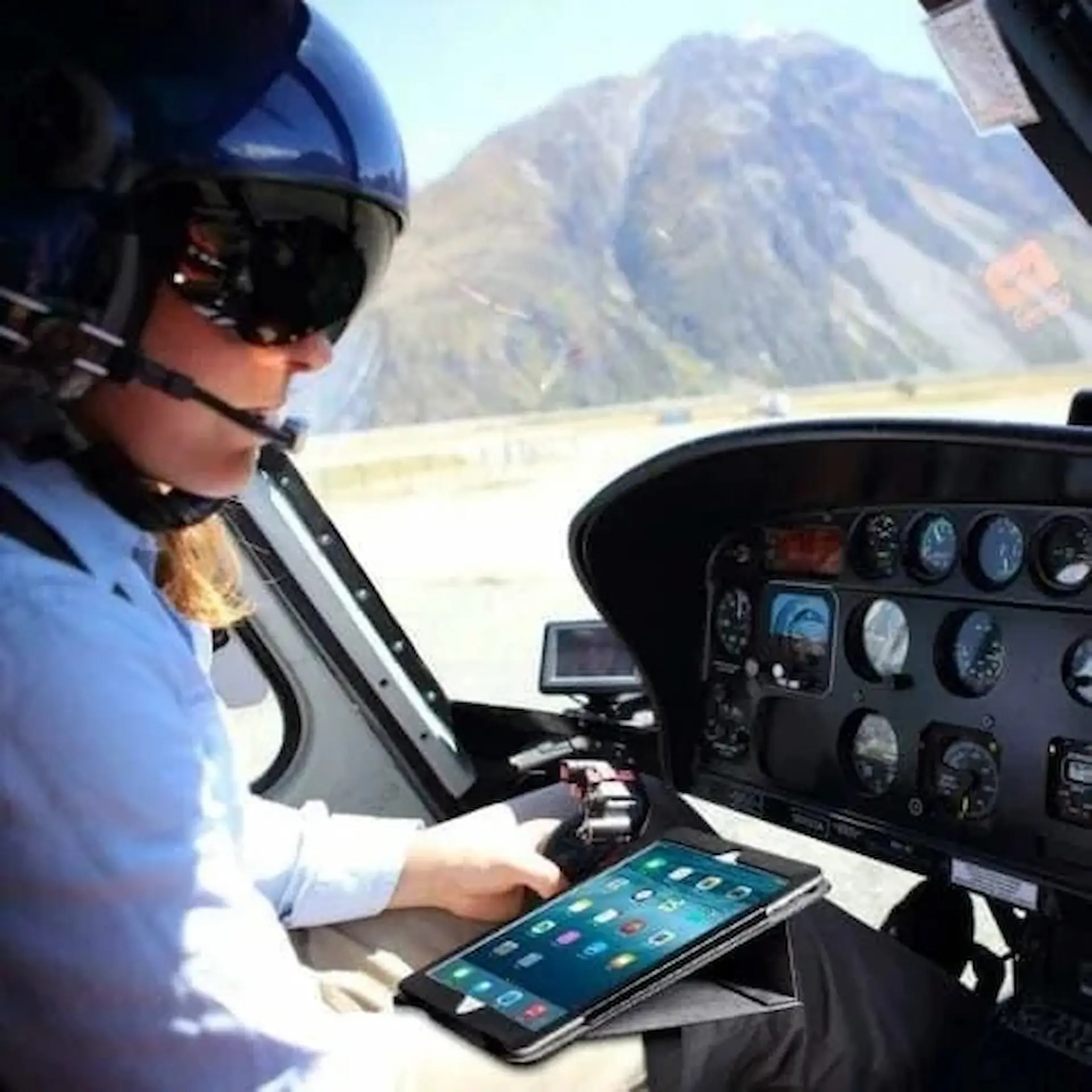
So, you’re in poor visibility on the taxiway at a busy airport, waiting on your slot time for an IFR departure to arrive at a distant airport equipped with conventional navaids only. You’re at Max All Up Weight with pax and cargo ( the stuff that pays the bills). You’ve enough fuel to meet the demands of alternates and weather, but if you delay too long on the ground, it’s back to the apron to top up again with all the commercial and crew duty period problems that entail. As you start to move off after the traffic in front of you, you get a caution light that your number one side FMS has failed. You need to know quickly if you can proceed with taking off. What AI product can help you here?
Web Manuals Amelia AI was launched in April 2024. Richard Sandström, Chief Technical Officer of Web Manuals Publication, describes it as follows:
We launched our first Web Manuals Amelia AI function in April 2024. The AI-search tool supports pilots and crew to efficiently source
information from within their digitized operational and safety manuals, making it faster and more intuitive than ever.
Users can pose questions to Amelia and get descriptive answers, helping them quickly make safe and well-informed decisions while avoiding
costly diversions and delays. The system seamlessly integrates natural language processing to speed up research work. For example, it can
help pilots to understand the cause of aircraft faults, assisting them when making airworthiness decisions.
The first version uses English natural language sentences as input. That said, we’re working on developing new versions which will expand the number of language options available. It will also be able to respond to voice demands as well as haptic input.
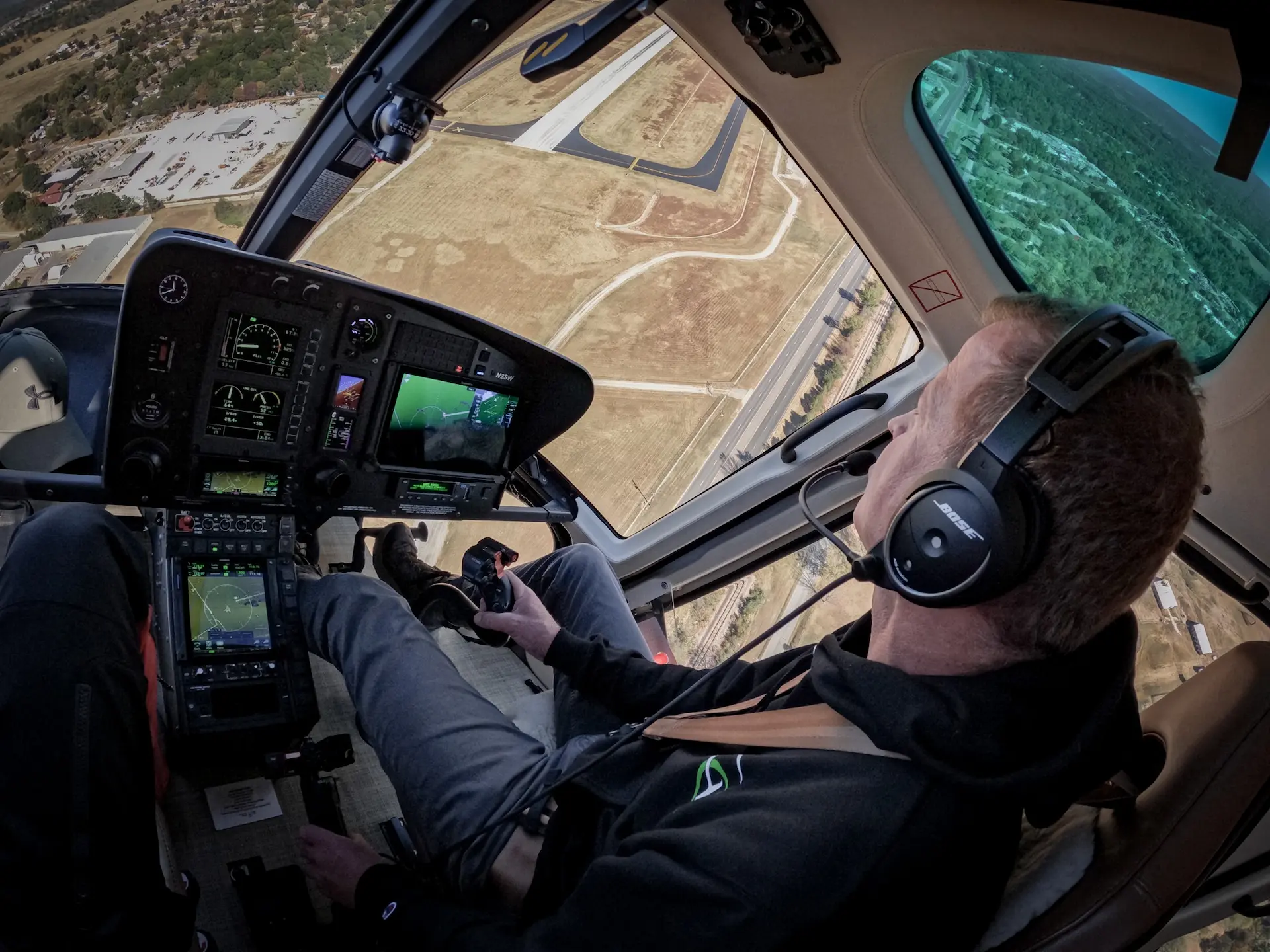
Using Plain Language
What this means for our scenario above is that the pilot can type in plain language, ‘Can I take off with one FMS?’ and receive an answer derived from the customer’s own MEL. In this case, our crew can likely proceed on their way so long as they don’t require an RNP approach at their filed alternate, and we have a little win for safety, scheduling and commercial efficiency all in one go. Safety as the pilot’s head isn’t dragged down for an extended period to consult the MEL, scheduling as slot times at a busy airport may be hard to get and commercial efficiency as no one likes delays/unhappy customers/extra fuel burned for no revenue.
Nevertheless, due diligence requires us to dig deeper to fully understand new and upcoming products. Potential pitfalls need to be avoided in product design, or at least those of which the operator needs to be aware.
AIs, like pilots, need training before they are set loose upon the world. This occurs when the AI is fed vast amounts of data, which is then analysed, allowing predictions to be made. These are then validated or tweaked (sometimes with human interference, sometimes not), and the results are refined through successive training rounds. Securing a fully trained product saves the operator from being exposed to the kinds of mistakes that anybody or anything in training makes in the early days, regardless of their ultimate potential. As an example, see some of the purported results ascribed to ChatGpts various maths tests… For our example:
Amelia is already a trained base model with natural language understanding. It generates responses in accordance with your documentation and will always give citations and sources from where it found the result. In future, we plan to train new models that are specific for the aviation industry. However, it’s unlikely that these models will be used for documentation search but rather used by our editors and administrators to empower and simplify their daily work.
Potential Pitfalls
So far so good, but one of the fears of a learning model is that it can pick up bad habits from its user, i.e if I personally stop asking for important information, will it stop providing it? Adapting to user behaviour is acceptable in a creative AI, but if it reinforces blindspots in pilots, that’s a different issue:
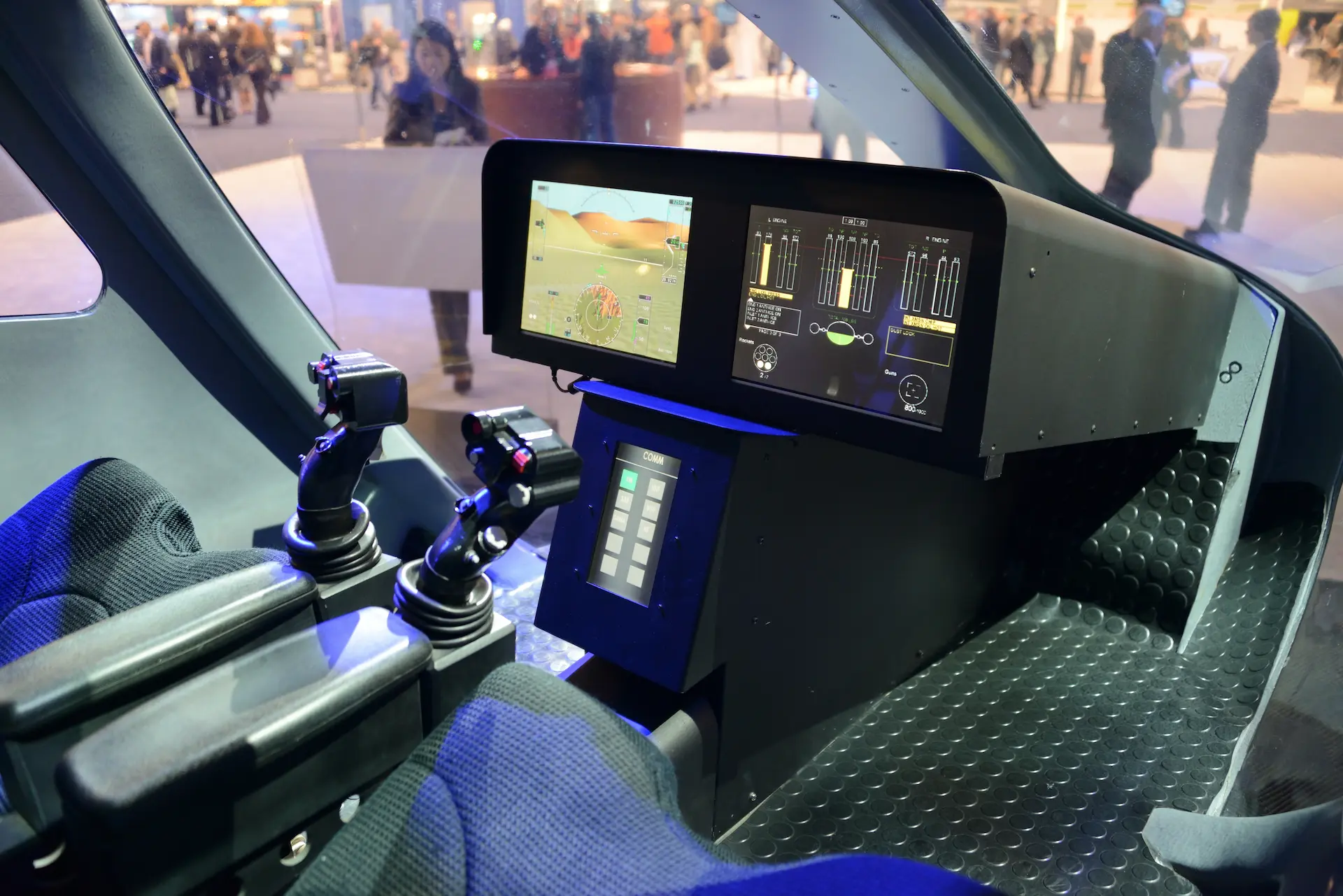
We decided not to develop a system that adapts to specific user behavior because of the associated risks. That said, we plan to evolve our product in future to offer users the option to manually promote certain results. This means that users will be able to report irritant results and further improve the AI system.
Controlling for user input in this way is beneficial, and also leads to the next area of concern – model decay. Model decay occurs when either reality changes from the data entered, i.e. new regulations are released but not incorporated, or when our interpretation of data changes over time, but the previously labelled data is not updated. So, for example, if approaches to a helipad using NVG were now considered as Performance Class 1, but no one told the AI, then it may give you bad information if you ask it, ‘can I fly HEMS to a helipad at night with a patient onboard?’. I asked Richard how Model Decay is controlled on an ongoing basis:
We continuously upgrade the model when new updates are available. We also offer the option for users to share feedback if results are irrelevant, making sure to evolve Amelia to best suit customer requirements.
As regulations change and documents are updated, Amelia can use new manual revisions as a basis for its searches. At this stage of enquiry we can be happy that our product is safe, but is it compliant? Specifically, we are asking questions in a ‘spoken language’ format and receiving a reply the same way, as opposed to the exact text that the national authorities have rubber-stamped and approved – is this a problem?
Web Manuals stores hundreds of digital manuals related to aviation safety and compliance. Our Amelia function only responds to queries related to content already stored in an operator’s existing documentation library. It provides a summary of the text, along with a reference of exactly where the information came from. It does not provide answers without giving you three different sources or citations from within your own documents.
Amelia represents a good example of how to introduce an AI into the aviation environment properly – it is iterative in that future versions may be more capable but it is being based on a cautious foundation, and it is controlled in that it is designed to reject bad habits and remain up to date with each new integration of data. While it does leave room for development, sensibly, it has stayed clear of critical systems. Speculatively, I asked if future versions may be used in a challenge and response manner to read out checklists:
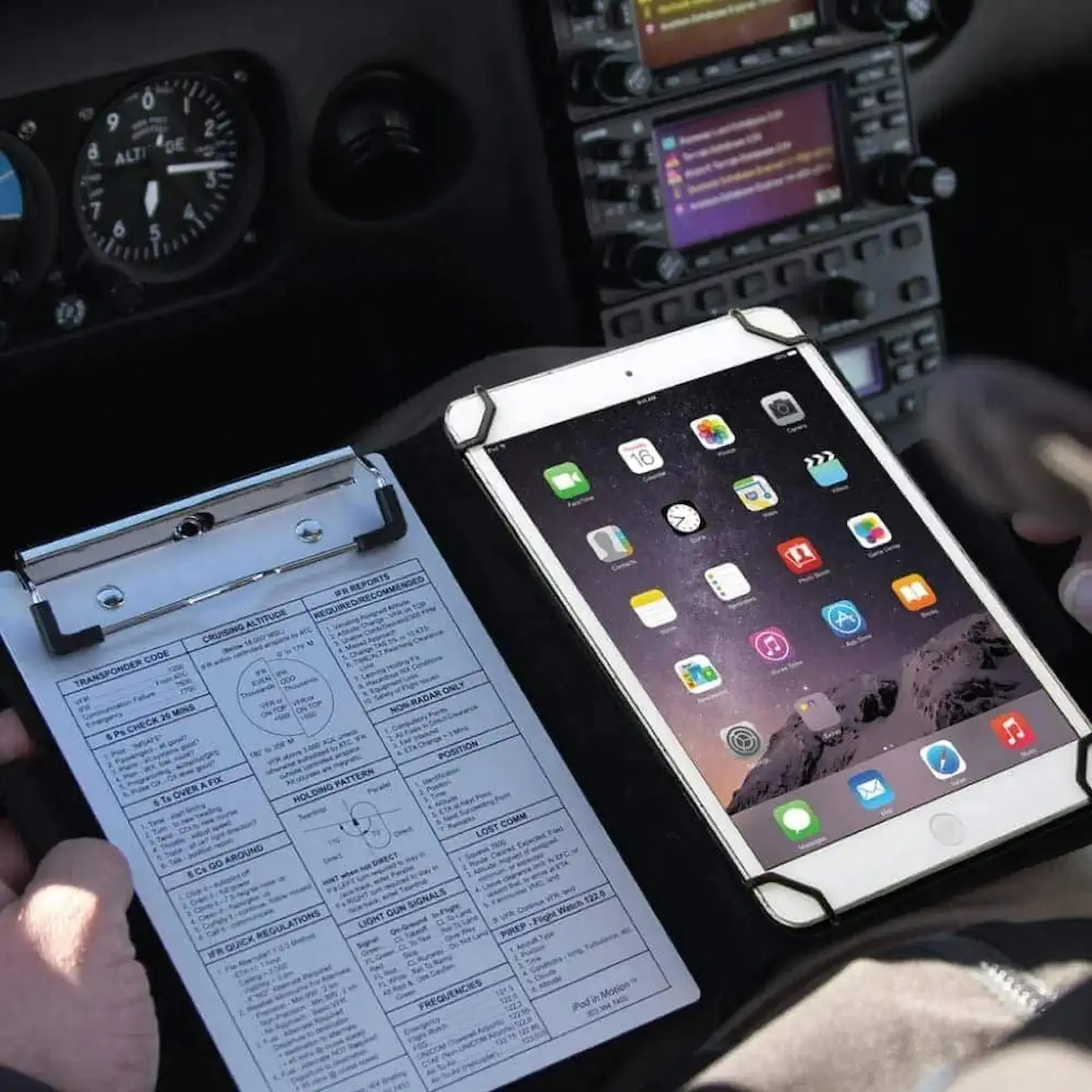
While Amelia provides a summary of results from within your documents, human oversight is still important and we encourage users to read the actual text from the citations provided. But, as AI technology improves, developing Amelia to read out checklists, for example, is certainly something we would consider.
Going forward, this area seems to be of particular use in single-pilot operations. I would suggest that it is also a new area for CRM Trainers to explore in depth before introduction – much as there is a proper time to be reading checklists and a time to just look out the window and fly, there will be a time when your AI can help you and a time when excessive use will cause distraction. Identifying these strengths and weaknesses should undoubtedly lead to exciting times in multiple training departments and sim sessions in the future.
‘You might develop a very sophisticated algorithm that appears to work every time. But in the end, is it certifiable?” - The Flight to Safety-Critical AI, Will Hunt UC Berkely CLTC White Paper Series
Amelia may be a product that has gone about things the right way, but more globally, there have been concerns about introducing AI into aviation in a manner that is as safe as any other part of the industry. The Centre for Long Term Cybersecurity (CLTC) at UC Berkely published a white paper focussing on some of the perceived risks associated with AI in general, and also the challenges to creating certifiable AI products to an aviation standard.
Collaborative
Reassuringly, they found that evidence of a race to the bottom was limited and that in fact there were signs that the opposite was the case:
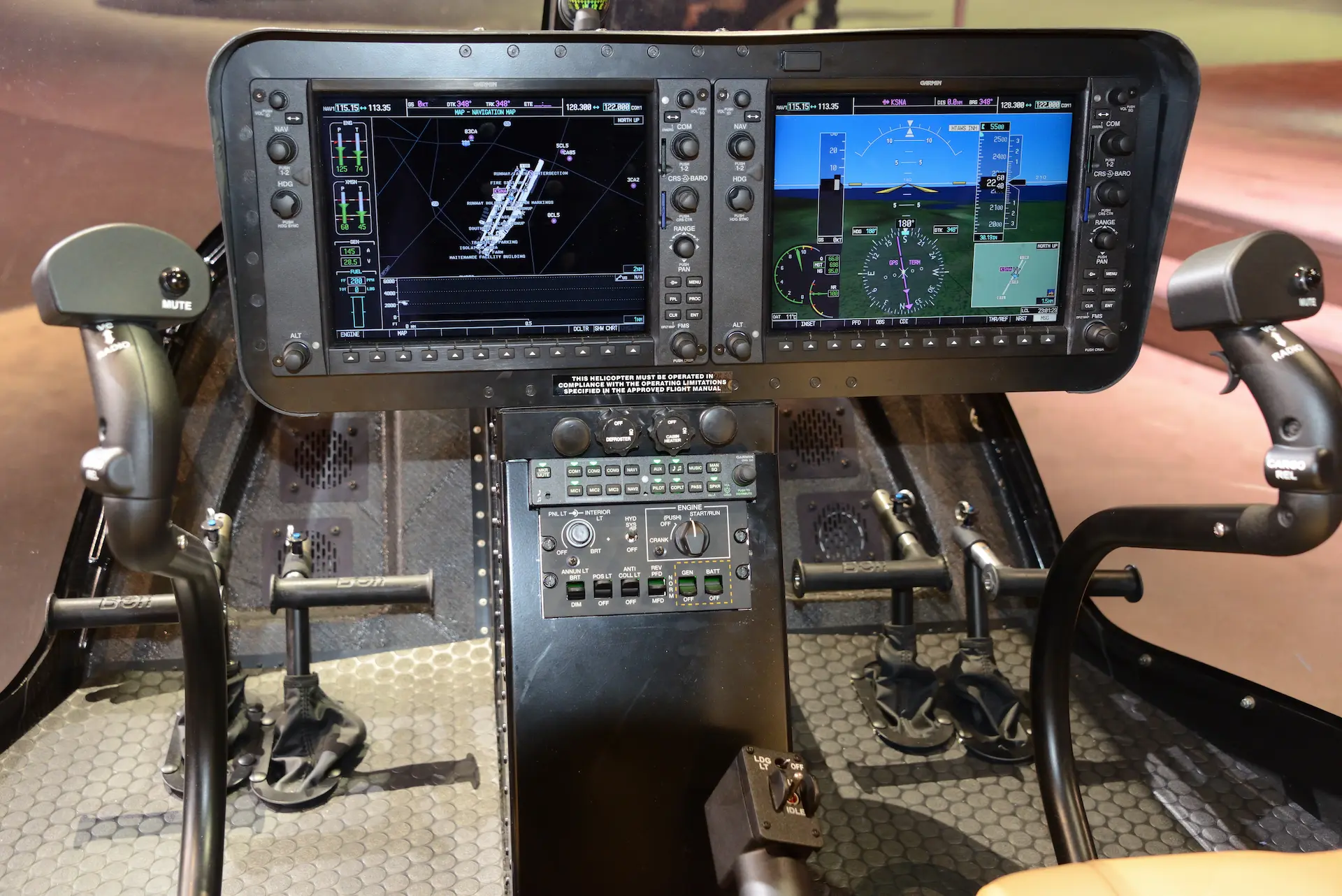
‘Industry experts report that representatives from firms, regulatory bodies, and academia have engaged in a highly collaborative AI standard-setting process, focused on meeting rather than relaxing aviation’s high and rising safety standards.’ - The Flight to Safety-Critical AI
In the area of safety-critical systems (your run-of-the-mill customer service chatbot may be different), all parties have recognised the serious consequences of failure to produce reliable results. However, there are still remaining factors inherent to AIs writ large that make certification difficult.
Firstly, AI as a concept is relatively new and aviation regulations precede the existence of AI products, so there is scant information available on which standard, if any, they are required to meet in most countries. As a result, the testing of the safety case of each individual application is in many cases, being delegated to the firms producing them. While they are at the moment pursuing a safety-conscious approach to development, independent oversight will be an essential part of this sector of the industry going forward; one needs only look at the 737 Max to see what happens when firms and regulators become too complacent in that regard.
The other difficulty highlighted in the report is described as the opacity of the technology – it is not easy for regulators to probe and test the logic of the algorithms that are employed in complex AIs, especially where a sign off is required on a machine that will keep learning through exposure to new data and usage. Consequently, the opinion of the experts interviewed in the white paper was that AI that interacts directly with safety critical systems in the aviation industry is more or less non-existent at this time, and that we are potentially decades away from anything we would recognise as a revolutionary change.
For now, it seems that products like Amelia, which assist the pilot with non-critical functions are much more likely to become a feature of our jobs than any more advanced system is to replace us entirely. The deliberate and cautious approach being taken by firms and regulators alike should serve to provide confidence in the products that have already made it through the system.